Building statistical machine translation based on sample bilingual text corpus
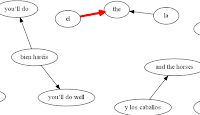
Training a weighted graph between the source and destination words and phrases in a sample bilingual text corpus yields an acceptable statistical machine translation dictionary. Following up on my previous post about language features , I felt that the simple sentence to sentence translation yielded too few translations, even though they were correct. I wanted to build something that creates a much bigger dictionary, even if it's noisier: false positives are still better than no translation at all. Larger graph Using the same sample, Don Juan from the Gutenberg project and mapping the Spanish source to the English translation I used a different approach this time: map each word and phrase in the source sentence to every word and phrase in the target sentence, adding more and more weights to transitions that keep appearing in the corpus. To quickly illustrate the idea, let's consider the following input and output sentence where each letter represents a word: a b c